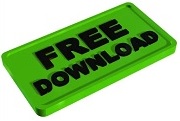

The unweighted pair group method phylogenetic tree resulting from the data of 68 isolates with the inter-PBS amplification DNA profiling method based on interspersed retrotransposon element sequences confirmed the differentiation of AGs with a higher resolution. The most prevalent anastomosis group (AG) was AG 4 (84%), which was subdivided into AG 4 HG-I (81%) and AG 4 HG-III (3%), followed by AG 5 (10%) and AG-A (6%), respectively. Based on the sequence analysis of the internal transcribed spacer (ITS) region located between the 18S and 28S ribosomal RNA genes and including nuclear staining, these 124 isolates were assigned to multinucleate Rhizoctonia solani, and eight were binucleate Rhizoctonia. This confirms that the comparisons are working with our multiprocessing and partial function.A total of 132 Rhizoctonia isolates were recovered from red cabbage plants with root rot and wirestem symptoms in the province of Samsun (Turkey) between 20. You’ll see that for the first third of the data (the one with 1/5 probability of being a 1) you can see that there is a peak with a Jaccard’s similarity score of 0.2 (20%). Attempting to accomplish the above task in a loop caused my computer to crash entirely (blue screen/frowny face), but if you are brave then you should try on a subset of data and see how long it takes.īelow is the result. You’re likely to come across datasets with more features and more observations. This is after multiprocessing and on 300k observations with 100 features.
#Jaccard coefficient xlstat code#
The above code takes almost a minute (~50 seconds). from functools import partial import multiprocessing as mp partial_jaccard = partial(jaccard_score, target) with mp.Pool() as pool: results = pool.map(partial_jaccard, ) Let’s see how many observations overlap with our target and by how much! But first, let’s make use of the multiprocessing package and create a partial function to compare several observations to the target in parallel (this is a huge time and memory saver). The first third has a probability of (1/5) of being 1, the second third a probability of (2/3), and the last third a probability of (1/2). This is mainly for the purposes of the example, but you can see how this can extend to other use-cases.Ī huge array of 300k observations is created with binary value data (1s and 0s) to stand in for indicator features or dummy variables. Imagine a basket that has bought every item available on your web store and you want to see which observations are closest to it. Our target is one observation where all the features are set to 1. Measure Distance with Jaccard’s and Parallel Processing import numpy as np import pandas as pd x0 = np.random.choice(, size=(100000,100), p=) x1 = np.random.choice(, size=(100000,100), p=) x2 = np.random.choice(, size=(100000,100), p=) colnames = X = pd.DataFrame(data = np.stack().reshape(300000,100)) X.columns = colnames target = np.ones(100).astype(int) Now that we’ve seen this metric under a simple case, let’s apply it to a larger dataset. This makes sense when under certain circumstances.) (Further be aware that some people make the distinction that the element 0 shouldn’t be included at all in the calculation. I personally prefer the similarity score offered in scikit-learn, but it’s important you are aware of the difference. One shows the dissimilarity and the other shows the similarity. The jaccard_score function returns the opposite: it’s the number of elements shared between the first two rows. Notice how the Jaccard function returns the number of elements not shared between the first two rows. The first row will be the observation we wish to compare to. Let’s check out a simple example: from trics import jaccard_score from import jaccard x = ,] print(x), , ] jaccard(x,x) 0.33 jaccard_score(x,x) 0.66 In our case, the denominator is the size of the either set, so we can also say that this similarity score is the number of shared elements divided by the number of elements that could be shared. So, when comparing two sets (which can be an array, a series, or even a vector of binary values) the numerator is the count of elements shared between the sets and the denominator is the count of elements from both sets.
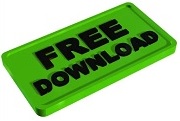